From Data to Discovery: How Structured Problem Solving Executes Analysis to Uncover Insights and Trends
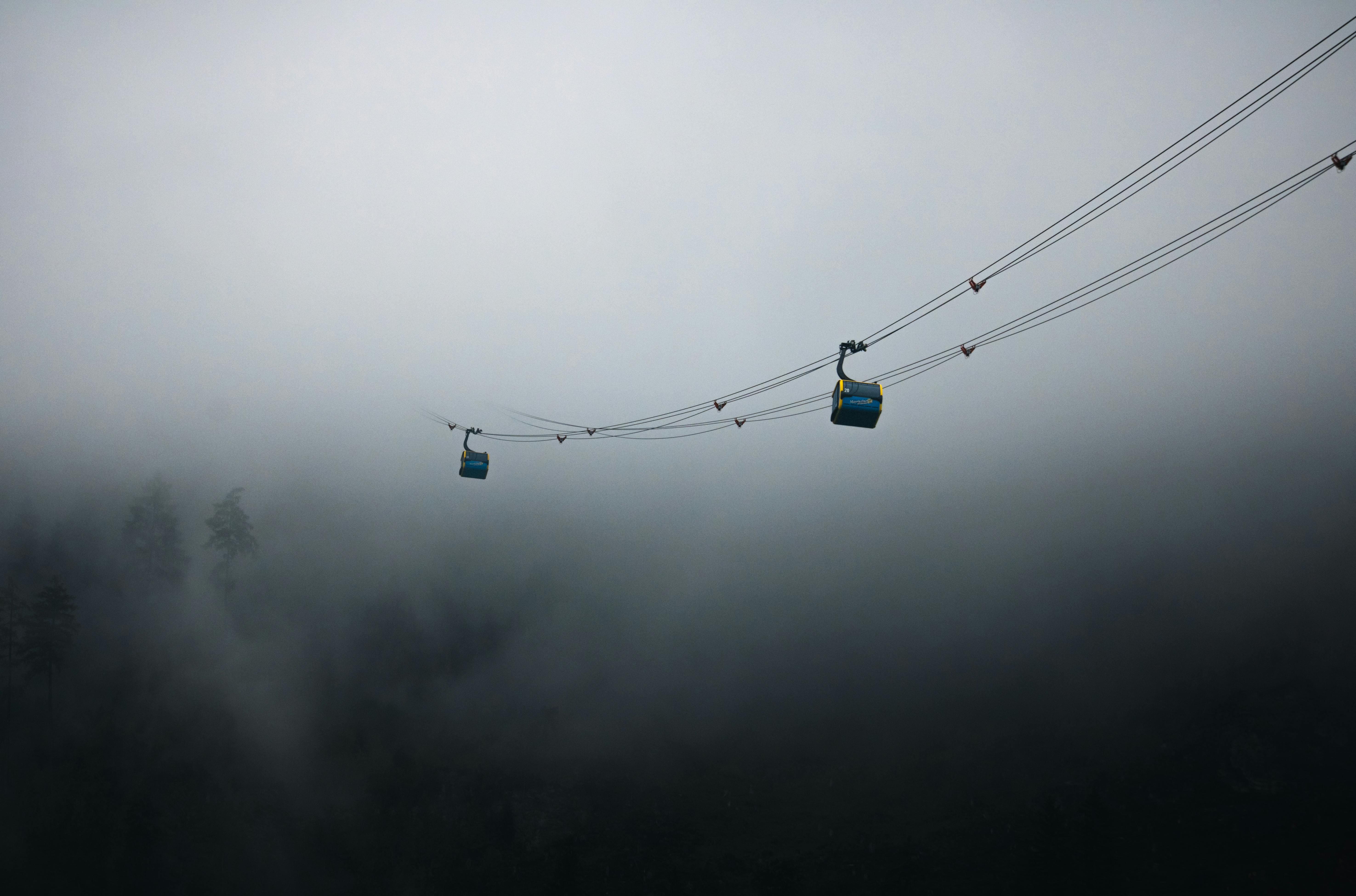
Despite the abundance of data available to organizations today, extracting meaningful insights remains a significant challenge for many. Teams often find themselves overwhelmed by fragmented datasets, unsure of where to begin or how to arrive at answers that drive action.
A structured approach directly addresses these risks by guiding analytical efforts through a repeatable, goal-oriented process. By following a clear sequence—preparing the analysis, processing the data, identifying trends, and interpreting results—organizations can transform raw information into strategic insight.
This article outlines how adopting a disciplined, step-by-step methodology enables teams to move from reactive data reviews to proactive, insight-driven discovery.
Prepare the Analysis
Effective analysis begins with a clearly defined objective. Prior to data acquisition or manipulation, teams must establish a focused business question or hypothesis. Whether investigating shifts in customer behavior, diagnosing performance issues, or testing assumptions, this central question serves as the anchor for all subsequent work.
Key preparation steps include:
- Identifying the business problem or hypothesis
- Clarifying the scope and intended outcomes
- Defining relevant metrics and KPIs
- Aligning stakeholders on goals and expectations
- Validating and securing high-quality data sources
This early alignment is crucial for ensuring buy-in and the relevance of the analysis to strategic objectives. When all parties understand the purpose and parameters, teams can avoid misalignment and accelerate decision-making later in the process.
Equally important is articulating the broader context—why the question matters and what decisions hinge on the outcome. A well-prepared analysis is not just a technical task; it's a strategic exercise that shapes focus and ensures impact.
Process the Data Raw data is rarely analysis-ready. It must be cleaned, formatted, and standardized to ensure analytical accuracy. Data processing transforms inconsistent or incomplete information into a reliable foundation for meaningful insights.
Typical processing activities include:
- Removing duplicates and correcting anomalies
- Filling or appropriately handling missing values
- Harmonizing definitions across datasets (e.g., consistent timeframes or classifications)
- Structuring the data for usability (e.g., tagging, normalizing, aggregating)
Every transformation or assumption should be documented to maintain transparency and enable reproducibility. This documentation also builds confidence in the results and facilitates review across technical and business audiences.
Insufficient attention to data processing can lead to flawed conclusions and, ultimately, misguided business decisions. Investing in data integrity upfront pays dividends in the credibility and utility of the final insights.
Look for Trends and Patterns
Once the data is structured, analysis moves into exploration. The goal at this stage is to identify trends and patterns that help answer the original business question—not simply to describe what the data says, but to connect it to organizational objectives.
Common exploratory techniques include:
- Descriptive statistics to summarize performance
- Data visualizations such as trend lines, heat maps, and bar charts
- Segmentation across time periods, customer types, or product categories
- Hypothesis testing to validate or disprove assumptions
This hypothesis-driven approach prevents unfocused exploration and ensures resources are directed towards the most promising areas of inquiry. By exploring within a strategic frame, teams can avoid chasing data points that lack relevance or impact.
It is also essential to select the appropriate analytical tools and techniques depending on the type of data and the question at hand. Whether leveraging statistical models, dashboards, or comparative analyses, the right method sharpens the accuracy and applicability of findings.
Ultimately, trends become meaningful only when they’re tied to action. Identifying a seasonal dip in engagement or a regional performance gap is valuable only when it points toward a change in strategy or execution.
Interpret the Results
The final phase translates analysis into insight. This involves interpreting what the data means for the business, not just what it shows.
Key activities include:
- Synthesizing findings into clear, focused takeaways
- Exploring underlying drivers and contributing factors
- Evaluating the reliability and business relevance of the conclusions
- Communicating insights in a way that supports decisions
- Presenting insights as actionable recommendations for stakeholders
Effective communication involves tailoring the level of detail and presentation format to the specific needs and interests of each stakeholder group. What a C-suite leader requires may differ from what a functional team needs—successful analysis adapts accordingly.
Precision and clarity are essential at this stage. Overcomplicating the narrative can dilute impact; underexplaining can leave insights vulnerable to misinterpretation. The goal is to tell a compelling story—backed by data—that enables confident action and measurable results.
Conclusion
Structured analysis is a business-critical capability. By methodically preparing, processing, exploring, and interpreting data, organizations elevate the value of their analytics function—from reporting to real discovery.
This disciplined approach empowers teams to deliver insights that are not only accurate, but also timely and relevant. In fast-paced environments, the ability to generate clarity from complexity is a distinct competitive advantage.
Smarter decisions, faster execution, and greater alignment all stem from structured analysis done well.
Next Steps
Empower your team to drive data-driven results.
Explore our Structured Problem Solving course to enhance your team’s analytical capabilities, sharpen bias-mitigation techniques, and master a practical framework for delivering effective, insight-led outcomes.